Table Of Content
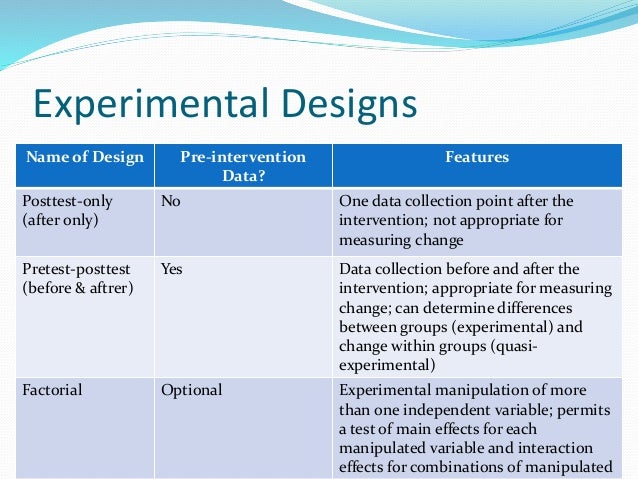
A quasi-experiment, on the other hand, does not depend on random assignment, unlike an actual experiment. However, even with random assignment, this research design cannot be called a true experiment since nature aspects are observational. Researchers can also exploit these aspects despite having no control over the independent variables. This research design is common in laboratory and field experiments where researchers control target subjects by assigning them to different groups. Researchers randomly assign subjects to a treatment group using nature or an external event or situation.
Examples of quasi-experimental designs
The leaders attended a six-week workshop on leadership style, team management, and employee motivation. Since every grade had two math teachers, each teacher used one of the two apps for three months. They then gave the students the same math exams and compared the results to determine which app was most effective.
STRATEGIES TO STRENGTHEN EXTERNAL VALIDITY
Obtaining pretest measurements on both the intervention and control groups allows one to assess the initial comparability of the groups. The assumption is that if the intervention and the control groups are similar at the pretest, the smaller the likelihood there is of important confounding variables differing between the two groups. This design involves the inclusion of a nonequivalent dependent variable (b) in addition to the primary dependent variable (a). Variables a and b should assess similar constructs; that is, the two measures should be affected by similar factors and confounding variables except for the effect of the intervention. Taking our example, variable a could be pharmacy costs and variable b could be the length of stay of patients. If our informatics intervention is aimed at decreasing pharmacy costs, we would expect to observe a decrease in pharmacy costs but not in the average length of stay of patients.
Causal Evidence Rating
In a study to determine the economic impact of government reforms in an economically developing country, the government decided to test whether creating reforms directed at small businesses or luring foreign investments would spur the most economic development. After a year, the researchers assessed the performance of each start-up company to determine growth. The results indicated that the tech start-ups were further along in their growth than the textile companies. The choice between these items (again, only one can be answered “yes”) is key to understanding the basis for inferring causality.
Differences Between Quasi-Experiments And True Experiments
Quasi-experimental designs enable you to investigate an issue by utilizing data that has already been paid for or gathered by others (often the government). Because the assignments are not random, it is harder to draw conclusions about cause and effect than in a real experiment. However, quasi-experimental designs are still useful when randomization is not possible or ethical.
Differences between quasi-experiments and true experiments
The design involved matching clinics by size and an inverse roll-out, to balance out the sizes across the four groups. The inverse roll-out involved four strata of clinics, grouped by size with two clinics in each strata. The roll-out was sequenced across these eight clinics, such that one smaller clinics began earlier, with three clinics of increasing size getting the intervention afterwards. This was then followed by a descending order of clinics by size for the remaining roll-out, ending with the smallest clinic. This inverse roll-out enabled the investigators to start with a smaller clinic, to work out the logistical considerations, but then influence the roll-out such as to avoid clustering of smaller or larger clinics in any one step of the intervention. It can be useful to obtain pre-test data or baseline characteristics to improve the comparability of the two groups.
Features of the Study
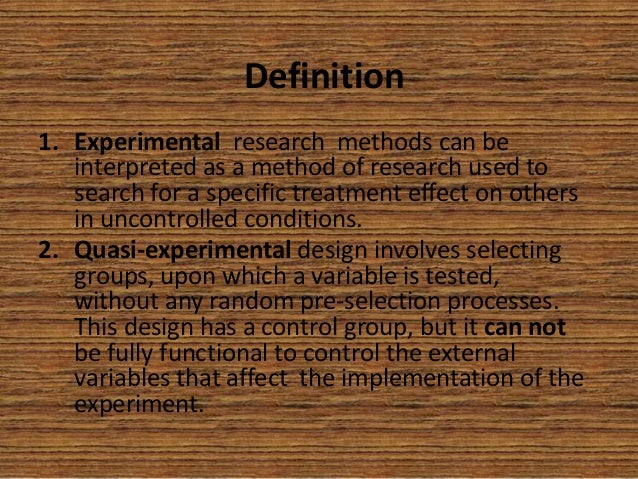
However, it doesn't use randomization, the lack of which is a crucial element for quasi-experimental design. This design involves collecting data on the dependent variable(s) over a period of time, both before and after an intervention or event. The researcher can then determine whether there was a significant change in the dependent variable(s) following the intervention or event. This design involves selecting two groups of participants that are similar in every way except for the independent variable(s) that the researcher is testing. The two groups are then compared to see if there are any significant differences in the outcomes.
Steps to improve brand health
The prefix quasi means “resembling.” Thus quasi-experimental research is research that resembles experimental research but is not true experimental research. Although the independent variable is manipulated, participants are not randomly assigned to conditions or orders of conditions (Cook & Campbell, 1979). Another alternative explanation for a change in the dependent variable in a pretest-posttest design is regression to the mean. This refers to the statistical fact that an individual who scores extremely on a variable on one occasion will tend to score less extremely on the next occasion.
Part 3: study design features and their role in disambiguating study design labels
One caveat is that if the intervention is thought to have persistent effects, then O4 needs to be measured after these effects are likely to have disappeared. For example, a study would be more convincing if it demonstrated that pharmacy costs decreased after pharmacy order-entry system introduction (O2 and O3 less than O1) and that when the order-entry system was removed or disabled, the costs increased (O4 greater than O2 and O3 and closer to O1). In addition, there are often ethical issues in this design in terms of removing an intervention that may be providing benefit.
Can a quasi-experimental research employ multimethod approach? - ResearchGate
Can a quasi-experimental research employ multimethod approach?.
Posted: Mon, 10 Feb 2020 08:00:00 GMT [source]
Quasi-experimental design, a fascinating method in the realm of research, offers a unique approach to uncovering cause-and-effect relationships. Unlike traditional experiments, where researchers randomly assign participants to groups, studies work with real-world constraints, employing non-random criteria for group allocation. This flexibility makes it a practical choice for exploring complex scenarios where strict experimental controls aren’t feasible or ethical. Question 7 asks about the variables that were measured and available to control for confounding in the analysis. The two broad classes of variables that are important are the identification and collection of potential confounder variables and baseline assessment of the outcome variable(s).
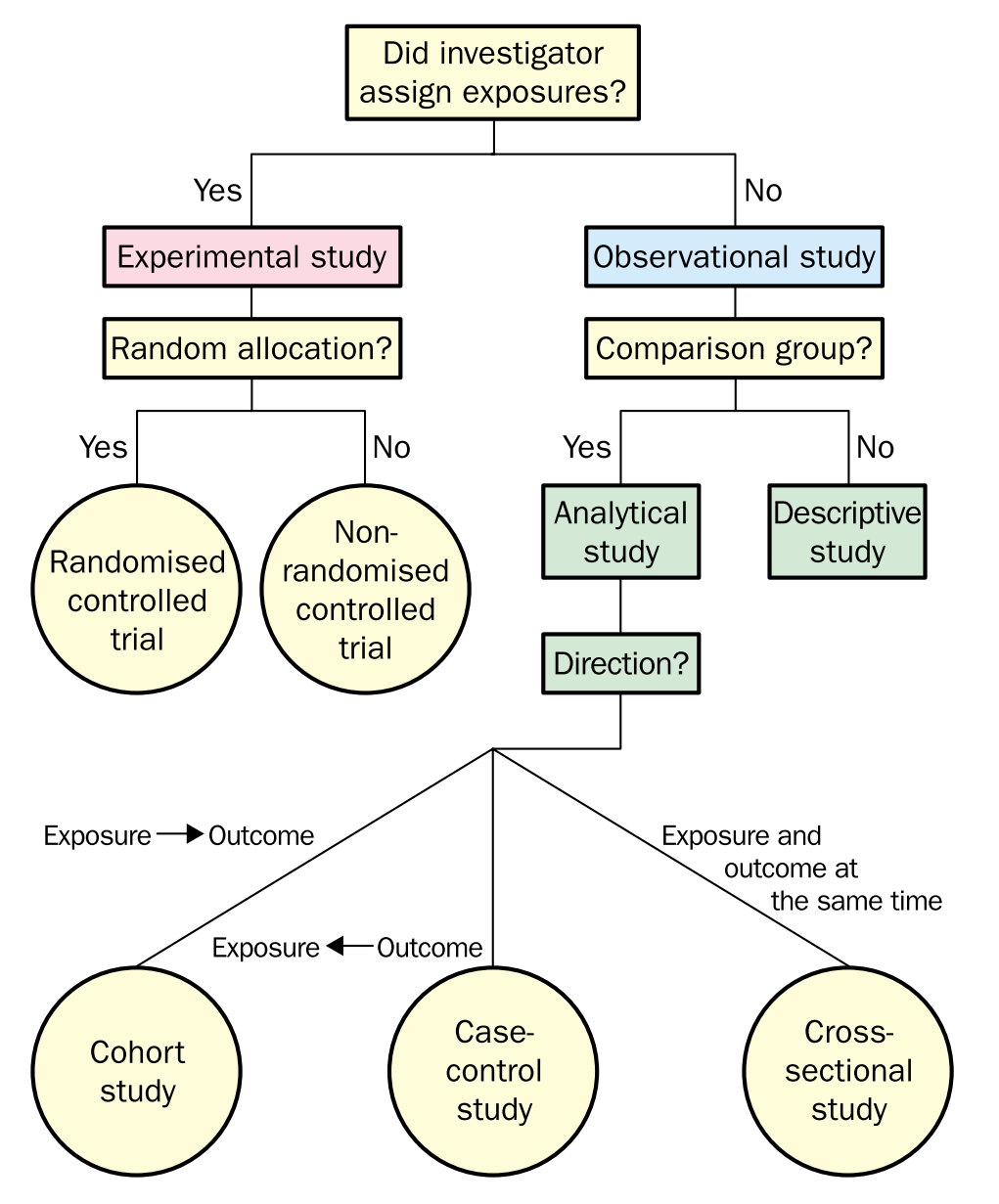
We conclude with a brief discussion of incorporating additional design elements to capture the full range of relevant implementation outcomes in order to maximize external validity. Finally, if participants in this kind of design are randomly assigned to conditions, it becomes a true experiment rather than a quasi experiment. In fact, it is the kind of experiment that Eysenck called for—and that has now been conducted many times—to demonstrate the effectiveness of psychotherapy. This would be a nonequivalent groups design because the students are not randomly assigned to classes by the researcher, which means there could be important differences between them. Because they allow better control for confounding variables than other forms of studies, they have higher external validity than most genuine experiments and higher internal validity (less than true experiments) than other non-experimental research. While both quasi-experimental designs and true experiments aim to uncover cause-and-effect relationships, they differ in terms of control over variables, randomization, and ethical considerations.
The order in which clusters receive the intervention can be assigned randomly or using some other approach when randomization is not possible. For example, in settings with geographically remote or difficult-to-access populations, a non-random order can maximize efficiency with respect to logistical considerations. Again, if students in the treatment condition become more negative toward drugs, this could be an effect of the treatment, but it could also be a matter of history or maturation. Some of the earliest CCT programs randomly assigned clusters (communities of households) and used longitudinal household survey data collected by researchers to estimate the effects of CCTs on the health of both adults and children [21]. The design and analysis of a cluster-randomized controlled trial of this kind is familiar to health care researchers [29]. The use of both a pretest and a comparison group makes it easier to avoid certain threats to validity.
“Resemblance” is the definition of “quasi.” Individuals are not randomly allocated to conditions or orders of conditions, even though the regression analysis is changed. As a result, quasi-experimental research is research that appears to be experimental but is not. This is a hybrid of experimental and quasi-experimental methods and is used to leverage the best qualities of the two. Like the true experiment design, nonequivalent group design uses pre-existing groups believed to be comparable.
For example, a research study shows that a new curriculum improved reading comprehension of third-grade children in Iowa. To assess the study's external validity, you would ask whether this new curriculum would also be effective with third graders in New York or with children in other elementary grades. If any substantial variations between them can be well explained, you may be very assured that any differences are attributable to the treatment but not to other extraneous variables. These pre-existing groups can be used to compare the symptom development of individuals who received the novel therapy with those who received the normal course of treatment, even though the groups weren’t chosen at random. As a result, in terms of internal consistency, quasi-experiments fall somewhere between correlational research and actual experiments.
Another important threat to establishing causality is regression to the mean.12,13,14 This widespread statistical phenomenon can result in wrongly concluding that an effect is due to the intervention when in reality it is due to chance. The phenomenon was first described in 1886 by Francis Galton who measured the adult height of children and their parents. He noted that when the average height of the parents was greater than the mean of the population, the children tended to be shorter than their parents, and conversely, when the average height of the parents was shorter than the population mean, the children tended to be taller than their parents.